An AI research assistant? This social scientist says it could be the future
Aritifical intelligence could transform some research methods, but managing bias in data remains a concern
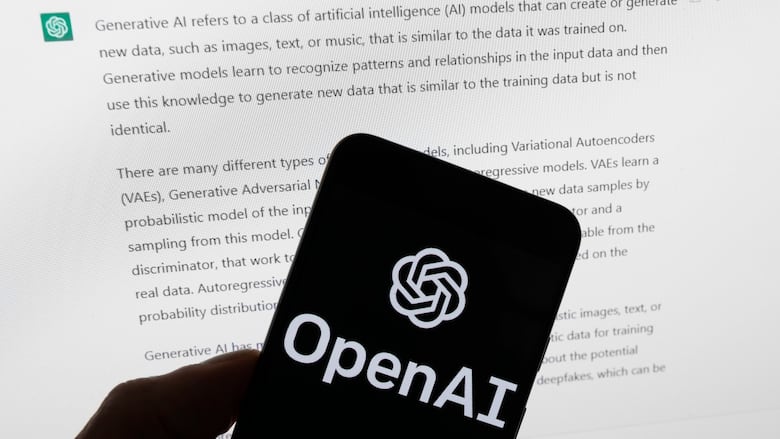
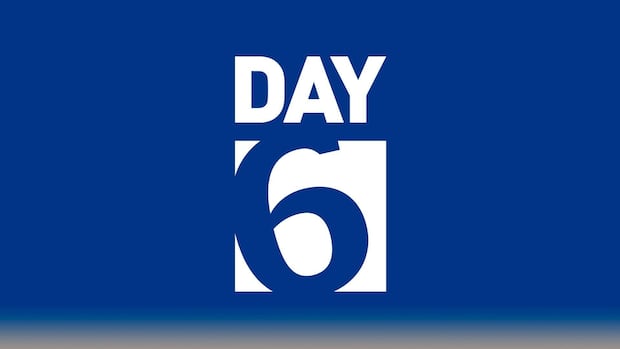
Igor Grossmann sees a future where social science research could be completed with artificial intelligence.
The University of Waterloo psychology professor says that AI could help researchers classify data, write code and, potentially, stand in as a research assistant. In some cases, AI could even substitute for human participants.
Researchers from Canadian and U.S. universities, including Grossmann, published an article in the journal Science last month surveying ways in which AI could change traditional social science research methods and replace human participants in some studies.
They point out that large language AI models "can represent a vast array of human experiences and perspectives" and could model human-like responses in surveys, represent opposing viewpoints in policy analysis and even simulate human behaviour in high risk hypothetical scenarios, such as space travel.
Grossmann spoke with Day 6 guest host Amil Niazi about what AI could offer the field moving forward and addressing key concerns with AI use. Here's part of that conversation.
What kind of reaction do you get when you tell people you're looking into how AI could replace humans in social science research?
It depends on whom I talk to. So some people, they are in disbelief and reject the idea outright. Others think that I propose some Orwellian future. And others are very curious or even tell me, "Well, this is old news, we knew that already," especially if I talk to some who are at the intersection of AI and computer science and behavioural sciences.
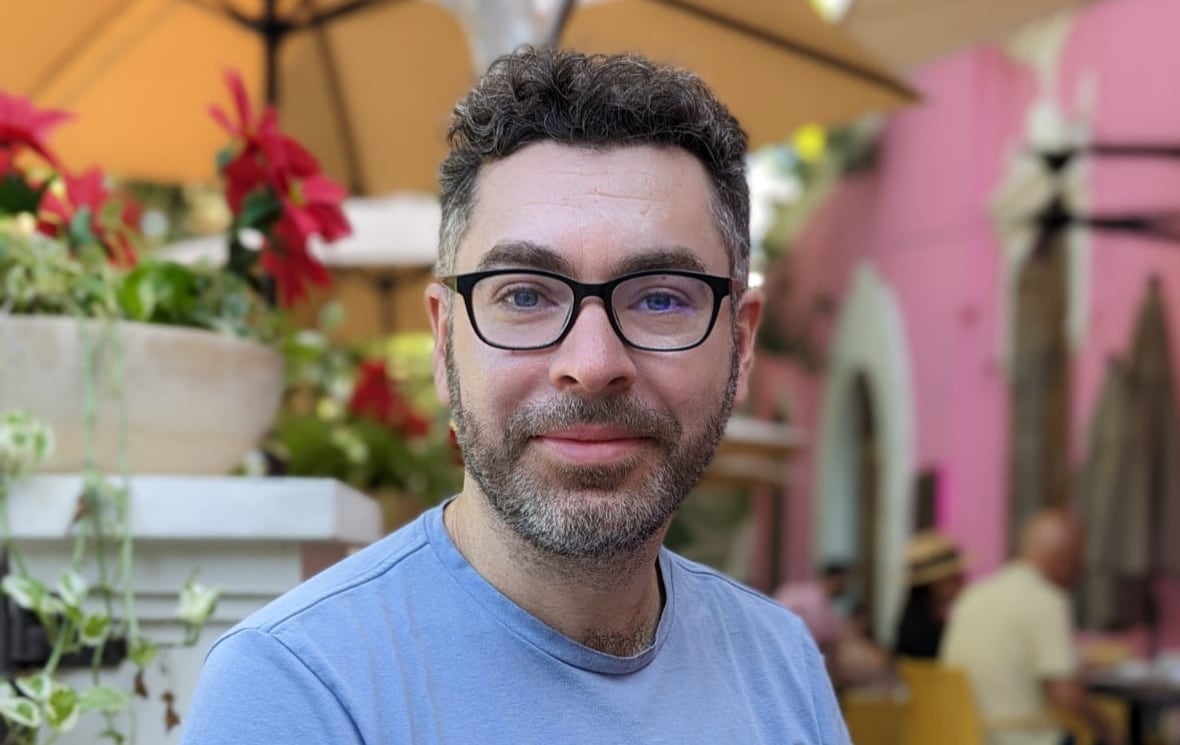
Scientists are already looking at using this to replace research assistants and other things. But what about actually using AI instead of human participants in this research? Can you walk me through what that would look like?
One possibility is you ask a model, like [OpenAI's] GPT-4, to create a range of responses to a particular question. And then you convert those responses to what a human would normally fill out on a scale from one to seven. And then you ask the model to do it again, again, again. Let's say you do it a thousand times. And then you'll basically have a silicon sample, as some people call it.
The critical part here is that what researchers often do is they first prime the model by creating a certain context. So the response should not be just a response, but it should represent the person from a particular demographic background, from a particular region, with a particular political leaning. So for instance, how ... a conservative Christian from Nebraska, who is in their 60s and who is male, would answer this question.
There are several studies that have been doing that, especially in [the] domain of political science. And there are some studies that do something different … they try to simulate responses on specific tasks, where previously human participants were used, to see how the model would respond on average, compared to humans.
And what's interesting there is that some of the biases that we also see in human responses, we also find in these online systems.
I'm glad you brought up bias, actually, because it is a big issue.... So I'm wondering if you have any concerns about, you know, where the data comes from and the training of the AI, and the quality involved?
I think most ... scientists, we are a little bit puzzled, on the one hand — excited and puzzled, so it's a lot of mixed feelings.
As a scientist, you want transparent communication of how certain models were trained, where the data is coming from, how the models were subsequently corrected through reinforcement learning, which is a particular strategy for this set of AI models.
What's interesting is that OpenAI, despite the name of the company, doesn't do any of that. It's a complete black box, be it in terms of the sources, the proprietary sources. We can guess where they're coming from, but we don't know 100 per cent.
So that makes it quite challenging. We can do some reverse engineering, but for many scientists like myself who argue for open methods, for open research practices, these models present an inherent challenge because we do know that in many ways they would either have biases built into them, because the culture is biased ... or there were biases creeping in when you tried to correct for those earlier biases by correcting them in the other direction.
Obviously you don't want the the model to be racist or xenophobic or chauvinist. But if you're a scientist and you're actually interested in studying those phenomena, if the model then has been corrected for those, then the outputs that you get are not representative of how humans appear to be responding to [a] certain set of questions in the society as is.
Why would someone want to use AI instead of humans?
I do think that for many [research projects] we should not use these types of models.
For instance, if you're studying a particular population that is not well represented in online texts or through other type of written media, using a model that tries to create some kind of a guess-based response of what that person, from that particular group, would be saying is probably strange, if not completely useless.
But I can totally see how some other groups that may be very well represented — and if you know that for this particular group, there's little room for bias or those biases, you may be testing for that. And [if] you assured yourself through additional research that those biases are not really an issue, you could simulate those responses at large and you could potentially test something that's very, very exciting.
Would you use this in your own research studies?
I'm very excited about using AI systems for helping [to] classify data, really as a research assistant. It creates a paradigm shift, I would say, so I'm very excited about that. In terms of [using AI in a] simulation, I'm not so sure.
I think there's some development that needs to be done, but I've already seen some interesting advances in this domain where an addition of AI on top of the agent-based model makes for much more realistic simulations of human responses.
Q&A edited for length and clarity