AI is reviewing scientists' old work and discovering things they missed
Scientists hope this AI system that turns words into math can help speed up the discovery process
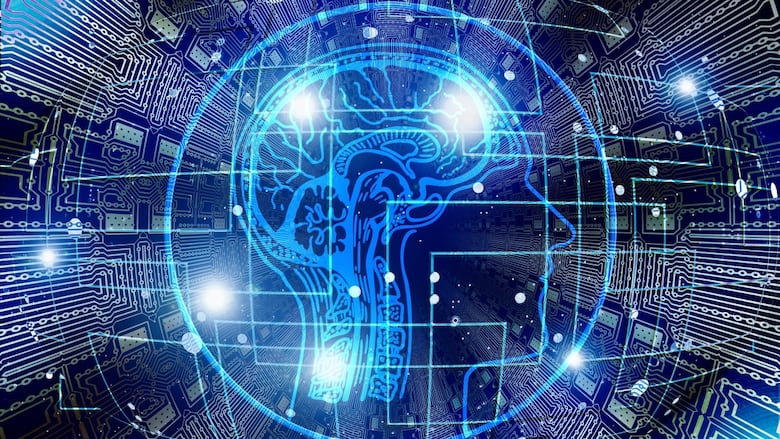
Originally published Nov.8, 2019
Material scientists from California have discovered a way to significantly speed up their discovery process, so much so that their newly developed artificially intelligent (AI) system has even made discoveries humans missed.
John Dagdelen, a PhD candidate in materials science and engineering at the University of California, Berkeley and a researcher at Lawrence Berkeley National Laboratory, told Quirks & Quarks host Bob McDonald that they trained the AI system on 3.3 million scientific paper abstracts to turn it into an extremely efficient kind of research assistant.
After Dagdelen and his colleagues trained the AI system, they asked it for a list of potential thermoelectric materials. Thermoelectric materials can convert heat into electricity.
Not only were the suggestions the system came up with better than a randomly picked material, but they were better than many of the thermoelectric materials known to science.
Extracting meaning from words
The AI system turns words into math by looking at how closely words appear together to figure out the word's meaning.
A classic example of this, which Dagdelen said gave him goosebumps when he first heard about it, is king - man + woman = queen.
To verify the accuracy of the system, they retrained it on material science abstracts that only went up to 2008 to see if it would give them predictions that have born out since then.
"We found that a number of the best thermoelectric discoveries over that time period were actually being predicted by the [AI] model years before they were actually predicted in reality."
Dagdelen and his collaborators are now synthesizing and testing materials the AI system suggested in hopes of turning predictions into real thermoelectric materials.