How AI researchers are teaching machines to collaborate like human coworkers
'It's a very close collaboration ... that really does require an ongoing process of feedback'
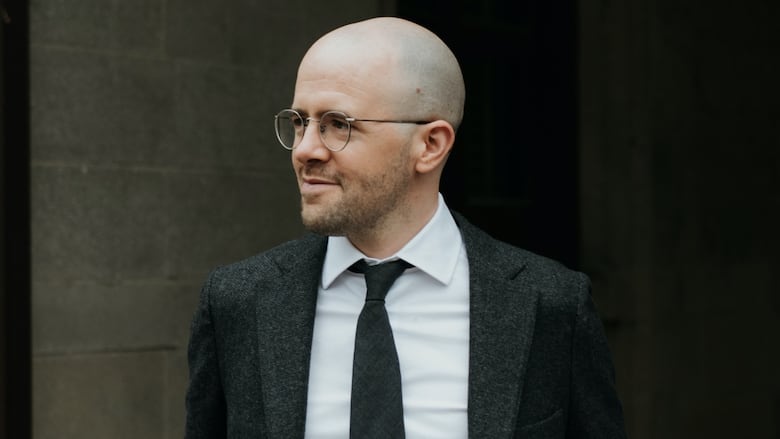
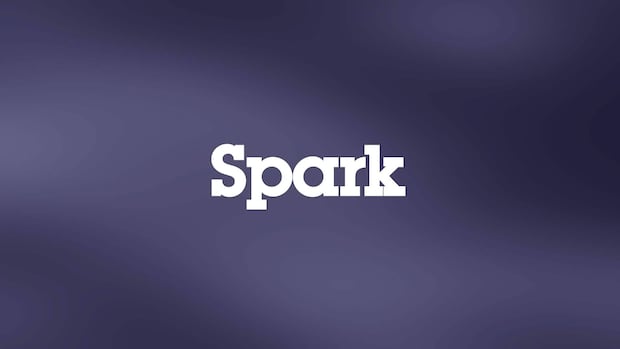
As machine learning systems are incorporated into more workplaces, our capacity to collaborate with robots has moved from the obscurity of sci-fi to the reality of 9 to 5.
In order for humans and artificial intelligence to work seamlessly together, researchers are working on how to teach machines to collaborate like human coworkers.
"Suddenly, we have to figure out what is going on under the hood when people move in a certain way, how do they expect that to be interpreted, because we now expect our robots to understand what we're trying to do in the same way," Brian Christian, a researcher focused on the human implications of computer science, told Spark host Nora Young.
Christian, author of The Alignment Problem: Machine Learning and Human Values, shared what we can learn about the future of our working relationships with artificial intelligence from the research that's happening right now.
Here is part of his conversation with Young.
You've written about training machine learning systems, not so much to achieve their objectives, but to help us to achieve our objectives...how to cooperate with us. Can you explain?
Yeah, I think we're really moving into a new phase in the field of machine learning, where we are treating the objective function itself as a machine learning problem that the computer has to infer. For me, the canonical example of this, or the foundational example of this was a study that was done between OpenAI and [Google's] DeepMind, on whether they could get a robot to do a backflip. And I love this example, because a backflip is the kind of thing that is very hard to specify with a mathematical formula. It's not clear at all how you would do that, but it's very easy to identify, if you see one, right? You know it when you see it.
They devised this procedure where their simulated robot would just wriggle around on the ground basically at random, and it would show you two video clips and say, "Which of these looks infinitesimally more like whatever you have in mind." And you think, "Okay, well, I guess that's sort of wriggling in the clockwise direction, so sure, okay." And it turns out that after about an hour of this … the system is able to do these beautiful gymnastic looking backflips and stick the landing.
To me that is a very, very big deal, because this is a proof of concept that we have the ability to convey these more ineffable human concepts, in this case, that kind of aesthetic concept for what a backflip entails. And we can get that concept out of our heads and into the machine without ever having to go through that step of manually turning it into a mathematical function.
As with that example, the backflip, does it typically rely on a human collaborating with the thing and kind of saying, "Yeah, that's a bit more like what I want you to do." Is that how it usually works?
Yeah, it's a very close collaboration. And, in fact, one of the things that the research team behind this discovered early on, is that it really does require an ongoing process of feedback. So if you give a bunch of data points at the very beginning, and then you tell the system to just kind of go off on its own and figure it out, you're not going to get a successful result.
What you need is, to do this kind of iterative feedback process where you give a little bit of feedback and then the system optimizes a little bit and then comes back to you and says, "Okay, now what do you think?" And you give a little bit more feedback. And through that process, that more interactive dialogue-looking process, that's how you end up getting there. And so I think that's a very telling statement, just about the sort of relationship that we're going to expect to have with these systems going forward.
How analogous is that type of process to what we know about the way humans learn from other humans to do things? Or [how] children learn from their parents to do things?
From a cognitive science psychology perspective, this ability to infer the goals of other people based on their behavior is absolutely foundational to human cognition. And it's potentially unique to humans, even compared to other primates. So there's been some really amazing work in developmental psychology by people like Felix Warneken, looking at how children — even as young as 18 months of age — can observe an adult who's reaching for an object that's out of reach, the children will realize that the adult needs help, and will spontaneously jump in to pick up the object and hand it to the adult.
I think that's very remarkable, because it shows that this ability to infer the goals and desires of other people is very hardwired. I mean, this is very early on in child development. And also the desire to help seems very hardwired, and potentially unique to humans as well. And so there's a lot still to be worked out in terms of where this capability comes from, how we're able to make this leap of understanding what people want just by observing what they're doing. But it is fascinating to me that we are now essentially replicating this capacity and this drive to help, in the machines that we're building.
Is there actually active work being done to research this — how, if a robot and a human are working on a physical object together, how those kinds of things can be taught [and] how that communication can be facilitated?
Yeah, this is absolutely an active area for academic roboticists at places like Berkeley, University of Washington, MIT. And in particular, this is of interest in a manufacturing setting. There's a real paradigm shift that's occurring right now, in industrial manufacturing. I think we're very much moving toward a system where, what manufacturers have in mind is a much more kind of elbow-to-elbow collaboration where there's no robot section and human section, there's just robots and humans fluidly moving in the same environment, working on something together, working on two different parts of the same object at the same time, or handing tools or pieces of this thing being constructed back and forth. And that sort of thing really requires a completely different approach and it requires that kind of gestural communication that humans … find so natural and effortless. Well, suddenly, we have to figure out what is going on under the hood when people move in a certain way, how do they expect that to be interpreted, because we now expect our robots to understand what we're trying to do in the same way.
If machine learning is increasingly being used in the workplace, what do you think that might look like? How will end users cooperate with an AI in practice, presumably after it's been trained?
I think it starts to take the form of something that looks like a dialog. And we've seen this with ChatGPT of course, that the original versions of GPT-3 and GPT-4 have a very specific structure, which is that they are built to auto-complete passages of text. And people who are very adept at working with them can find all sorts of creative ways to use that autocomplete ability to do various things from machine translation to programming and so forth. But it is not the natural way that humans … work with one another. It's [not] like,"Okay, Nora, here's a text. Can you complete this text?" Rather, it's a conversation, there are requests that are made, there are instructions given. And so I think that's been the real leap forward with ChatGPT, which is no more powerful than the underlying systems that it was based on. But it's much more user friendly, you might say, much more intuitive, because your interaction with it takes the form of a conversation.
Where do you think there might continue to be tensions in a working relationship with machine learning systems or even with robots?
When I think about the phrase, "the alignment problem," this is an expression that the computer science community has borrowed from economists. Economists in the '80s and '90s were writing about: how do we create a value aligned organization? How do we align the incentives of your subordinates with your goals as the manager — or whatever it might be. And I think that's a very useful metaphor to keep in mind as we think about our relationship with machine learning systems, because there is that question of, even if you can perfectly understand one another, are the incentives aligned?
And so that's a source of tension in human relationships. This comes through in the workplace all the time, where individual incentives might diverge from team incentives and so people end up kind of infighting within a corporation or something like that. I think that's going to end up being very significant when we think about our relationship with machine learning, and I think this is already the case. An AI assistant might perfectly understand what we want but still, in some fundamental way, it's trying to help me as the user, but it also needs to serve the business model of the company that's providing the system to me.
Q&A edited for length and clarity.